Why We Created ForecastOS
Before ForecastOS, I helped build a machine learning pipeline for a quantitative investment strategy at CPPIB, a large Canadian pension fund.
Perhaps unsurprisingly, the biggest challenge our team faced was: it's near impossible for one person to understand, analyze, audit, and backtest AI-powered financial forecasts.
If you're one of the lucky few who can, you cannot do so quickly, or with a single tool. It's a complex process by nature, with co-dependent datasets, features, forecasts, and portfolio optimizations / backtests. Furthermore, the complexity erodes productivity and prevents the involvement of non-technical colleagues. This results in fewer predictive ideas, sub-optimal forecast performance, reluctance to take risk / deploy capital, and very hard to manage projects.
We built ForecastOS to solve this problem. ForecastOS allows anyone to understand, analyze, audit, backtest, and (dare I say) manage their financial forecasts through a friendly UI without changing their current solution at all; our tools plug into the machine learning pipeline you already have and tie all of the associated pieces together!
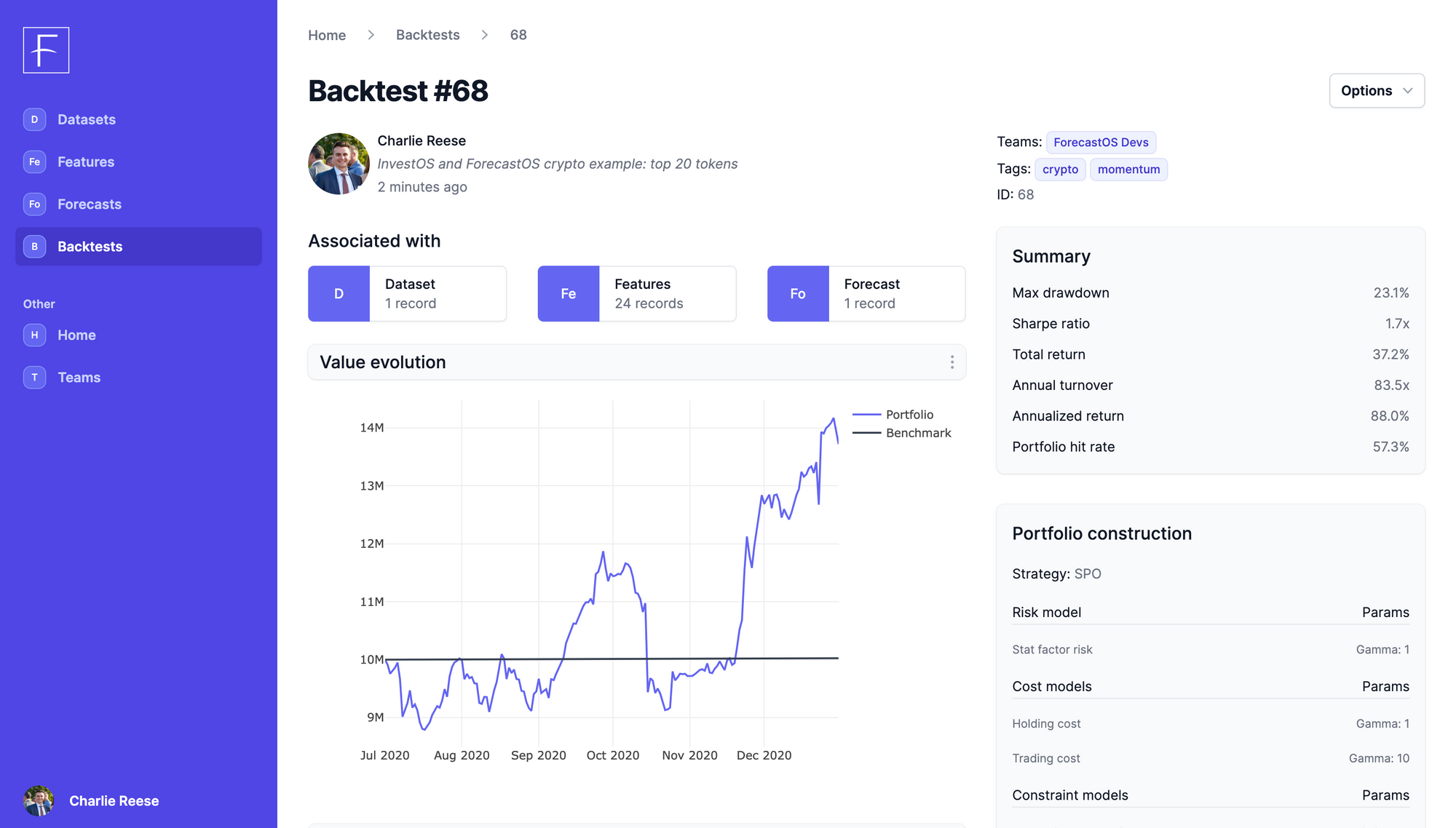
How did we do it? Underlying our solution are three core beliefs:
- All financial forecasts and associated backtests share the same core building blocks. With the right abstractions and visualizations, anyone can understand and contribute to financial AI
- More contributions yield better ideas (inputs), which yield better forecasts (outputs). Your ML engineers cannot brainstorm every possible predictive idea on their own. Find a way to invite others in
- All financial forecasts and associated backtests have unique requirements. Solutions must allow for infinitely flexible data engineering, forecasting, backtesting, and reporting. You can't expect anyone to use a rigid platform with unnecessary constraints, or expect them to change their implemented solution at all
We'll discuss each of our three core beliefs in our next article.
--
Looking to simplify your financial forecasts or to learn more? Be sure to subscribe to our blog and check out our demo!
Note: if you want to learn more about portfolio optimization and backtesting for quantitative stragies, check out our open-source module InvestOS, which integrates with ForecastOS!